Advances in how computers analyze and apply data have trickled down from Silicon Valley laboratories and into corporate legal departments worldwide, denoting a sprint toward the future rather than a slow crawl. One of its most practical and exciting use cases involves legal document machine learning and contract analysis. While there are plenty of reasons to use this technology right now, it is also imperative that companies understand where current limitations exist to avoid blind spots and maximize workflow efficiency.
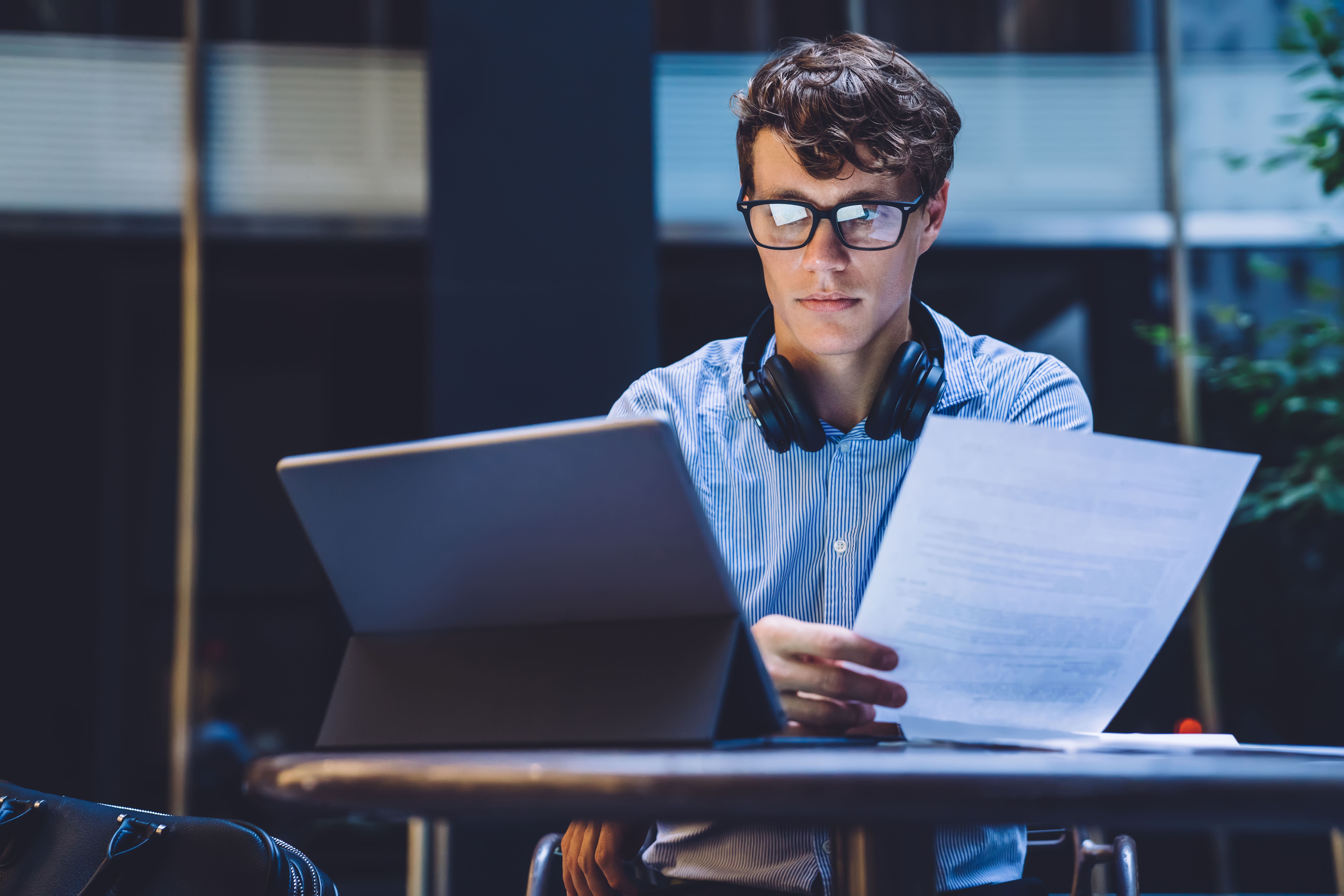
Why Invest in Legal Document Machine Learning Now?
High turnover rates in corporate legal departments have stretched resources thin. Although daily legal work can be more complex and include drafting and reviewing bespoke agreements, a good portion of it usually involves routinely reviewing high volumes of standard contracts with boilerplate provisions. And it seems there are never enough hours in the day to properly review and negotiate them all.
Tech-forward legal departments are leaning on platforms based on artificial intelligence (AI) and legal document machine learning to power through their review work. At some tasks, AI and machines are far more scrupulous than human beings, including the following:
- Sorting through data: Once a certain clause is deemed significant by a human reviewer or by its inclusion in a resource like an AI Digital Playbook, machine learning trains itself to remember and find similar clauses in future documents.
- Recognizing patterns: Attorneys can train systems to look for missing sections, incorrect words, vague language, and inconsistencies.
- Assigning context: Attorneys can configure AI platforms to add contextual notes for any flagged passages or recommendations, making negotiations much clearer and faster.
- Assessing risk: AI can review third-party changes to standard forms and provide risk assessments based on an AI Digital Playbook. For example, “red” issues can be automatically escalated to a legal professional for a governed response. “Yellow” issues can be assessed and self-negotiated with situationally correct guidance. And “green” issues can be automatically accepted and incorporated into the document.
- Reviewing and negotiating: Machines never tire or become distracted when performing tasks, so these systems are ideal for taking the first pass and catching common drafting errors.
- Standards compliance: AI and legal document machine learning help solidify company practices by applying a consistent set of standards to multiple agreements.
- Saving time: No single attorney can read, review, and redline an entire contract in five minutes or less, but machine learning-based solutions can.
- Focusing attention: Attorneys frequently lose time reviewing non-essential terms and, as a result, miss areas of negotiation that greatly impact the company’s bottom line. Machine system flagging can help reviewers bypass boilerplate issues and concentrate their time on the terms and issues that truly matter.
Attorneys spend a great deal of time combing through contracts line by line. As a result, they do not have much time to work on bigger picture reforms and client-side services.
Supervised vs. Unsupervised Machine Learning Contract Review
Two types of machine learning apply to legal document review platforms:
- Unsupervised machine learning leverages built-in algorithms to assess similarities, patterns, and differences. These systems do not require any human input or intervention. They are built on best practice guidance and proprietary data acquired by the company, as well as internal logic that dictates how the machine applies past experiences to present review work. In this way, a system can detect a missing termination clause or legal terminology that deviates from the legal playbook.
- Supervised machine learning allows users to essentially train the system. Not all legal document review platforms offer this level of sophistication, but this type of technology can transform how legal departments work. Rather than spending countless hours reviewing agreements, attorneys instead create complex rules to train the system to do the heavy lifting for them. For instance, an attorney could train the AI by writing specific rules, such as: “If X occurs, then Y.”
Workarounds To Machine Learning’s Limitations
Traditionally, hurdles to machine learning advancement have included:
- Lack of open source training data (as most legal data is confidential and proprietary)
- Language complexity (especially considering rapidly changing rules and legalese)
While machines cannot precisely understand causality, sophisticated technology combining machine learning with natural language processing (NLP) can apply linguistic intelligence to text analysis. As a result, AI-powered legal solutions can provide actual redlines and helpful suggestions that close legal loopholes and limit risk in future contract negotiations.
Machine learning was never meant to be a standalone technology. But when combined with AI, NLP, and attorney oversight, it can become a critical element of a legal team’s workflow by reducing disputes, compliance issues, and negotiating inconsistencies over time.